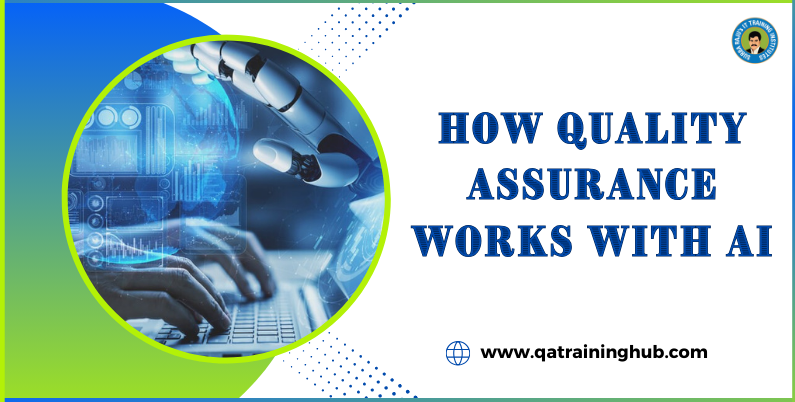
Unveiling the Collaboration: How Quality Assurance Works with AI
Quality assurance (QA) processes play a crucial role in maintaining high standards and meeting customer expectations.Since the introduction of artificial intelligence (AI), QA methods have changed a lot, using AI-powered tools and techniques to improve efficiency and accuracy.In this article, we explore how quality assurance works with AI, discussing its advantages, difficulties, and what the future holds.
Evolution of Quality Assurance:
Quality assurance has been a crucial aspect of manufacturing and software development for many years. Initially, QA involved manual testing, where human testers carefully examined products or software for defects or inconsistencies. Though it worked, manual testing was slow, demanded a lot of resources, and was susceptible to human mistakes.
As technology progressed, Automated testing became a practical choice instead of manual testing. Automation tools made it easier to run test cases, speeding up testing and boosting efficiency. Yet, automated testing still depended on predefined scripts and didn’t have the flexibility needed to deal with complex situations and changing software systems.
The Rise of AI in Quality Assurance:
Adding AI to quality assurance has completely changed how testing works. It’s way faster, more accurate, and can handle bigger tasks. AI-powered QA tools use smart algorithms and advanced analytics to study huge amounts of data, identify patterns, and make quick decisions as things happen.
One of the key advantages of AI in QA is its ability to perform predictive analysis, allowing organizations to anticipate potential issues before they become critical defects. By analyzing historical data and detecting patterns, AI can predict failure points, allowing proactive measures to be taken to reduce risks and ensure product reliability.
Key Components of AI-Powered QA:
Test Automation:
AI-powered test automation goes further than regular automation by using machine learning algorithms to create test scripts dynamically.These smart test scripts adjust to any changes in how the application works, making sure all areas are thoroughly tested and speeding up the time it takes to get the product to market. AI-powered test automation tools can also decide which test cases are more likely to fail, making testing more efficient and using resources better.
Intelligent Test Case Generation:
AI algorithms can examine system requirements, user stories, and past data to create test cases automatically. By understanding the application’s functionality and user interactions, AI-driven test case generation ensures thorough test coverage while minimizing manual effort.This method is especially useful in agile development settings, where the rapid delivery of high-quality software crucial.
Anomaly Detection:
AI-powered anomaly detection methods can spot when things aren’t going as expected in real-time. By continuously monitoring system metrics and user actions, these algorithms can find any unusual signs that might suggest there’s a problem or a security issue. Finding problems early helps QA teams investigate and fix them quickly,reducing their impact on the end-user experience.
Natural Language Processing (NLP) for Requirements Analysis:
Natural language processing (NLP) algorithms help AI systems understand and make sense of written requirements, user stories, and documents.NLP-powered QA tools make test planning and execution more precise by pulling out important details and recognizing connections.Moreover, NLP can automate creating test cases from written requirements,making testing smoother and reducing manual work.
Benefits of AI-Powered QA:
Improved Efficiency: AI-driven QA automation speeds up the testing process, allowing organizations to release high-quality products faster.
Enhanced Accuracy: AI algorithms can find small defects that human testers might miss, making the software stronger and more dependable.
Cost Reduction: By automating repetitive testing tasks and using resources better, AI-powered QA helps organizations save money on testing while still keeping quality high.
Predictive Maintenance: AI-based predictive analytics help in proactive maintenance, reducing downtime and improving the overall dependability of the system.
Scalability: AI-powered QA solutions can easily expand to manage big and complicated software systems,ensuring consistent performance in various environments.
Challenges and Considerations:
Although AI offers great potential for improving QA processes, implementing it comes with its own challenges and factors to consider:
Data Quality and Bias: AI algorithms require large volumes of high-quality data to train effectively. Making sure the data is accurate and avoiding bias in training sets is crucial to avoid getting misleading results and wrong predictions.
Interpretability: Some AI algorithms can be difficult to understand how they make decisions. Making sure AI models are clear and It’s important for AI models to be easy to understand for people to trust them and work with them effectively.
Security and Privacy: AI-powered QA tools could accidentally reveal private information or create security risks. Having strong security measures is crucial to protect data privacy and prevent potential threats.
Skill Gap: Using AI in QA needs specific skills like machine learning, data science, and software engineering. Closing the skill gap and giving enough training is important to get the most out of AI-powered QA.
Future Directions:
As AI technology advanced, the future of QA holds a lot of potential for new ideas and progress. Some trends and developments in AI-powered QA include:
Autonomous Testing: AI-powered autonomous testing frameworks can create their own test cases, run tests, and analyze results without needing humans to step in.
AI-Powered DevOps: Using AI in DevOps practices helps with continuous testing, deployment, and monitoring, making it quicker to deliver software and improving its quality.
Explainable AI: Creating AI models that are easier to understand and explain helps stakeholders trust the decisions made by AI.
AI-Driven Test Prioritization: Changing the priority of test cases based on insights from AI ensures that testing covers the most important areas and uses resources well.
Conclusion:
In conclusion, the integration of AI into quality assurance marks a big change in how testing works. At QA TrainingHub, we understand the importance of staying up-to-date with these changes. Our training programs provide professionals with the skills and How Quality Assurance Works with AI and also know-how to use AI-powered QA tools effectively.
As organizations aim to provide high-quality products and services in a competitive market, combining AI with traditional QA methods opens up unique chances for innovation and expansion. Embracing AI-driven QA helps businesses simplify testing, speed up product launches,and enhance customer satisfaction.
Join QA TrainingHub and know How Quality Assurance Works with AIÂ and start a journey of learning and growth as we discover the endless possibilities of AI in quality assurance. Together, let’s influence the future of QA and show the way for a smarter, stronger, and technology-driven future.